The platform belongs to those who work it:
The Amara case study
by David Rozas
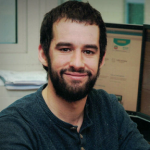
Co-authors: Elena Martínez Vicente, Paulo Colombo, Jorge Saldivar, Eve Zelickson
Data Range: 2018-20
Region: global
Methodologies
Social research
• Semi-structured Interviews
• Focus group
• Participant observation
• Documentary Analysis
Technology
• Ethereum
• Infura
• React
• IPFS
• Aragon/Aragon Connect
• The Graph
Design
• Lean Design
• Design Thinking
• Co-creation
• Workshops Prototype & Iteration
• User test
Related papers/preprints
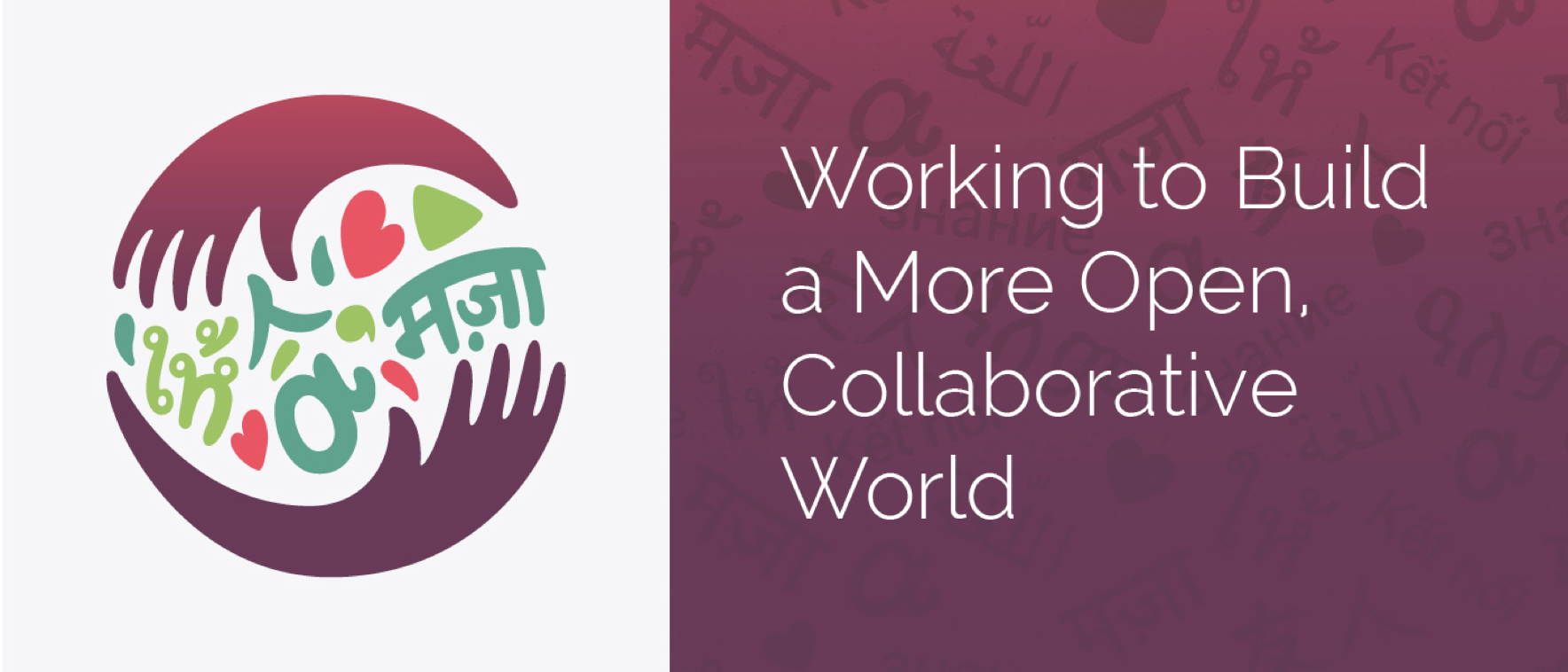
Amara is a project of the Participatory Culture Foundation (PCF), a nonprofit working to build a more inclusive media ecosystem since 2006. Amara is an open and collaborative subtitling platform [1]. Examples of organizations that use the Amara platform to create subtitles through volunteer engagement include Khan Academy, Scientific American and California Academy of Sciences [2]. As a research project, however, our focus is on the use of the Amara subtitling platform as an on-demand and paid service: Amara On Demand (AOD). AOD was launched in 2013 [3] as a result of the success of the Amara platform [1]. Over the past few years, AOD has grown from just a few linguists to more than nine hundred at the time of writing. The work of linguists in AOD is remunerated, in contrast to the volunteer subtitling supported by the Amara platform in the aforementioned case of Khan Academy. AOD is inspired by cooperative and commoning practices [4], presenting a remarkable contrast when compared with the market-based logic of other crowdsourcing platforms.
Why did we choose Amara?
We are framing AOD as part of the broader phenomenon of crowdsourcing. Nowadays collaboratively creating knowledge is the economy’s primary productive force [5,6], and digital platforms increasingly mediate contemporary working practices. On crowdsourcing platforms, work is “taskified” [7]. Entering receipts into expense reports, curating data to train artificial intelligence software, translating texts and tagging words and images are all examples of work that can be easily “taskified”. These tasks are carried out by an invisible workforce of “humans in the loop” [4]. Through platforms such as Amazon Mechanical Turk (AMT), this volatile and globally distributed crowd of workers with varying degrees of expertise and backgrounds [8] compete with each other to find tasks to work on.
Hansson et al. [9,10] explored the different modes of production mediated by crowdsourcing platforms by studying 21 different cases. Crowdsourcing platforms such as AOD and AMT were categorised as “Human Computing”, i.e. those in which “users do micro-tasks that do not require much expertise, such as transcribing audio and video files, translating texts, or tagging maps” [9].
To further our understanding of how crowdsourcing platforms might support social relationships in these contexts, instead of merely capitalizing on them [9], we decided to study, establish a collaboration with and develop a set of experimental tools with AOD. The strong cooperative values embedded in AOD [4] offer, in this respect, an opportunity to experiment with fostering more relational crowd models, in which the producer is also the owner of the means of production and the products created are an expression of self-realization [9]. AOD contrasts with platforms such as AMT [4], which understand workers as random passive objects from which tasks and items can be sourced [9].
Our vision: “The platform belongs to those who work it”
Our ultimate goal consists of experimenting with a gradual shift in decision-making power (governance) over the rules that determine how value is distributed to the members of crowdsourcing platforms. Our vision can be summarized by the motto: “The platform belongs to those who work it.”1
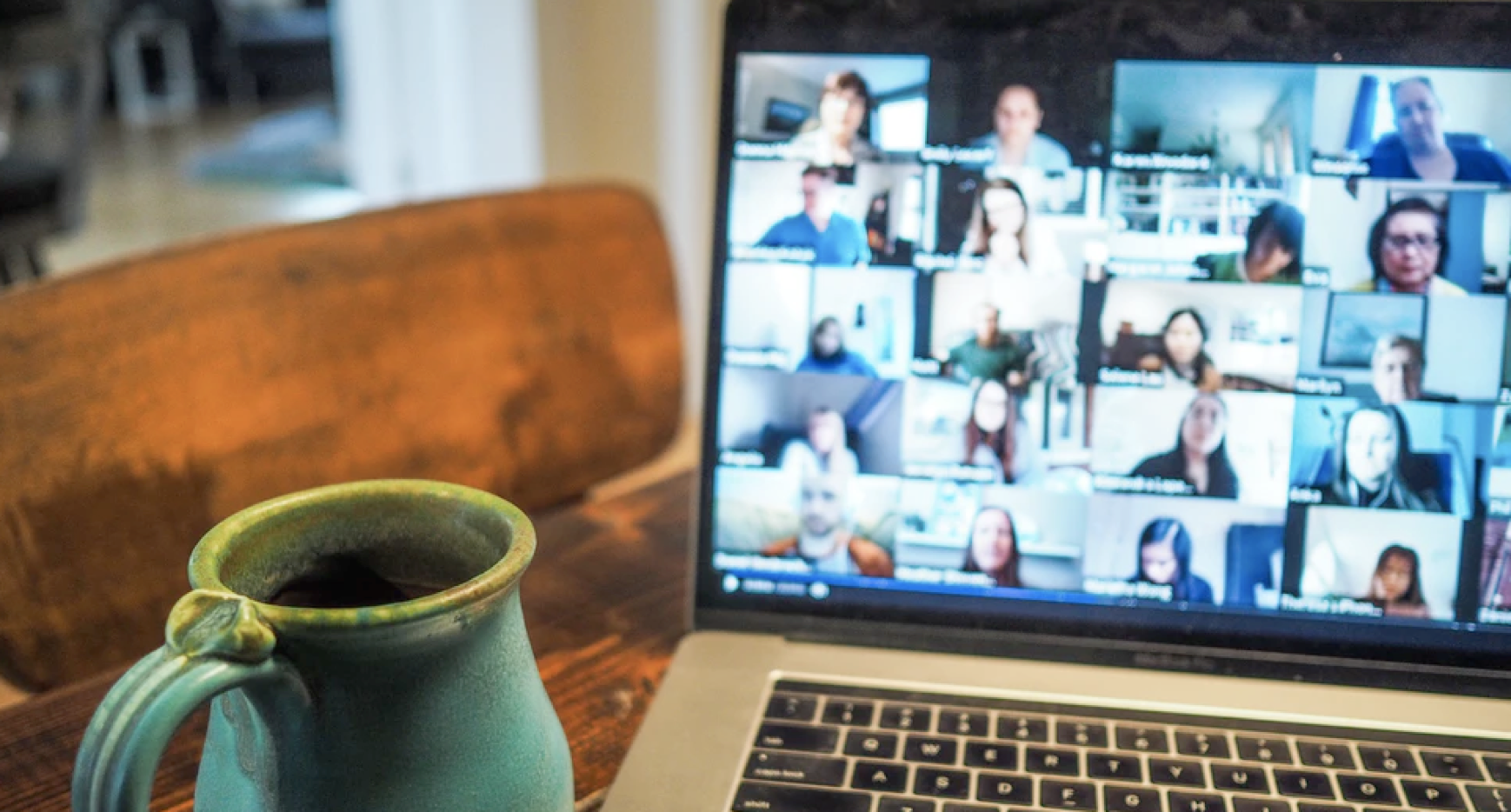
Photo by Chris Montgomery on Unsplash
We aim for this research to have an impact beyond this case study and to influence a broader range of areas of the collaborative economy. Our goal is to increase worker participation and ownership of the platforms that mediate their work. Similar decentralized platforms owned by worker cooperatives, which distribute tasks and value according to their own agreements, could be envisioned for a myriad of areas. An example might be a cooperative of taxi drivers whose organization is mediated by a blockchain-based platform that they control. The platform could distribute and monitor rides and payments according to rules defined by the workers within their specific context. Ultimately, we expect to explore and contribute to the development of worker-centric crowdsourcing platforms [7] and, more generally, to that of platform cooperativism [13] within the context of blockchain-based tools that embed commoning practices [14].
What are we experimenting with?
As a research group, we are experimenting with blockchain technologies to help collaborative economy organizations work in a more decentralized way. Our aim is to explore the potentialities and limitations of blockchain technologies to experiment with alternative value distribution models and more participatory decision-making mechanisms. In this case study, we first needed to identify the best point of intervention to do so.
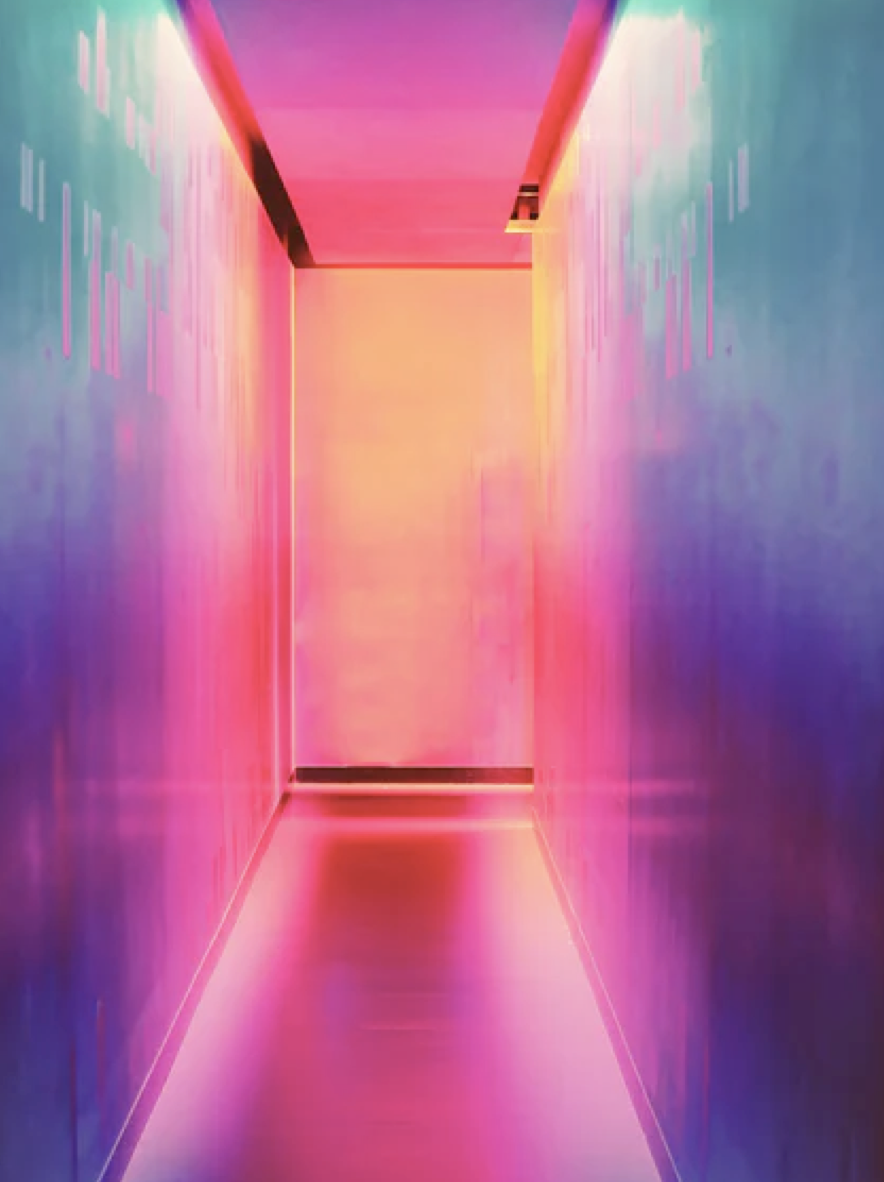
1. We have adapted this motto inspired by the motto coined by Teodoro Flores in the context of the Mexican Revolution (1910-1920): “La tierra es para quien la trabaja” (The land belongs to those who work it). The motto captures the revolutionaries’ vision for land reform compiled in the Plan of Ayala [11], written by Emiliano Zapata together with other Mexican revolutionaries [12].
Stage 1. In-depth analysis of AOD
Stage 1 (October 2018 – March 2019) focused on an in-depth analysis of the inner workings of AOD from the perspective of the linguists. From the onboarding process to daily operations, this first iteration of data collection and analysis provided a rich picture of the experiences, needs and workflow vision of participating AOD linguists. The research involved online participant observation and 15 semi-structured qualitative interviews. The selection of interviewees during this stage aimed to reflect the diversity of AOD linguists in terms of language group, experience level and degree of engagement. The primary outcomes during this stage of research were the mapping of AOD workflow and the identification of an initial set of communitarian needs, which led us to identify several points of intervention as potential candidates to experiment with the development of blockchain-based tools.
Mapping the Workflow
Groups organize themselves in AOD based on language direction, i.e. which languages they can translate to or from English. For example, if a customer requires a set of videos in German to be subtitled into Spanish, this will involve the groups German → English and English → Spanish.
Once a linguist is admitted into the community and agrees to the rules outlined in the Amara guidelines, they are added to their language team’s mailing list. Project Managers send job orders by email, which linguists use to sign up for tasks. These tasks are allocated on a “first-come, first-served” basis. Figure 1 and Table 1 provide an overview of the overall workflow in AOD, as identified and mapped by Zelickson [3] at the time2 the research was conducted (October 2018 – April 2019). While linguists do not have direct control over the creation and modification of organizational rules, they do help to enforce them when performing quality assurance on others’ work [3].
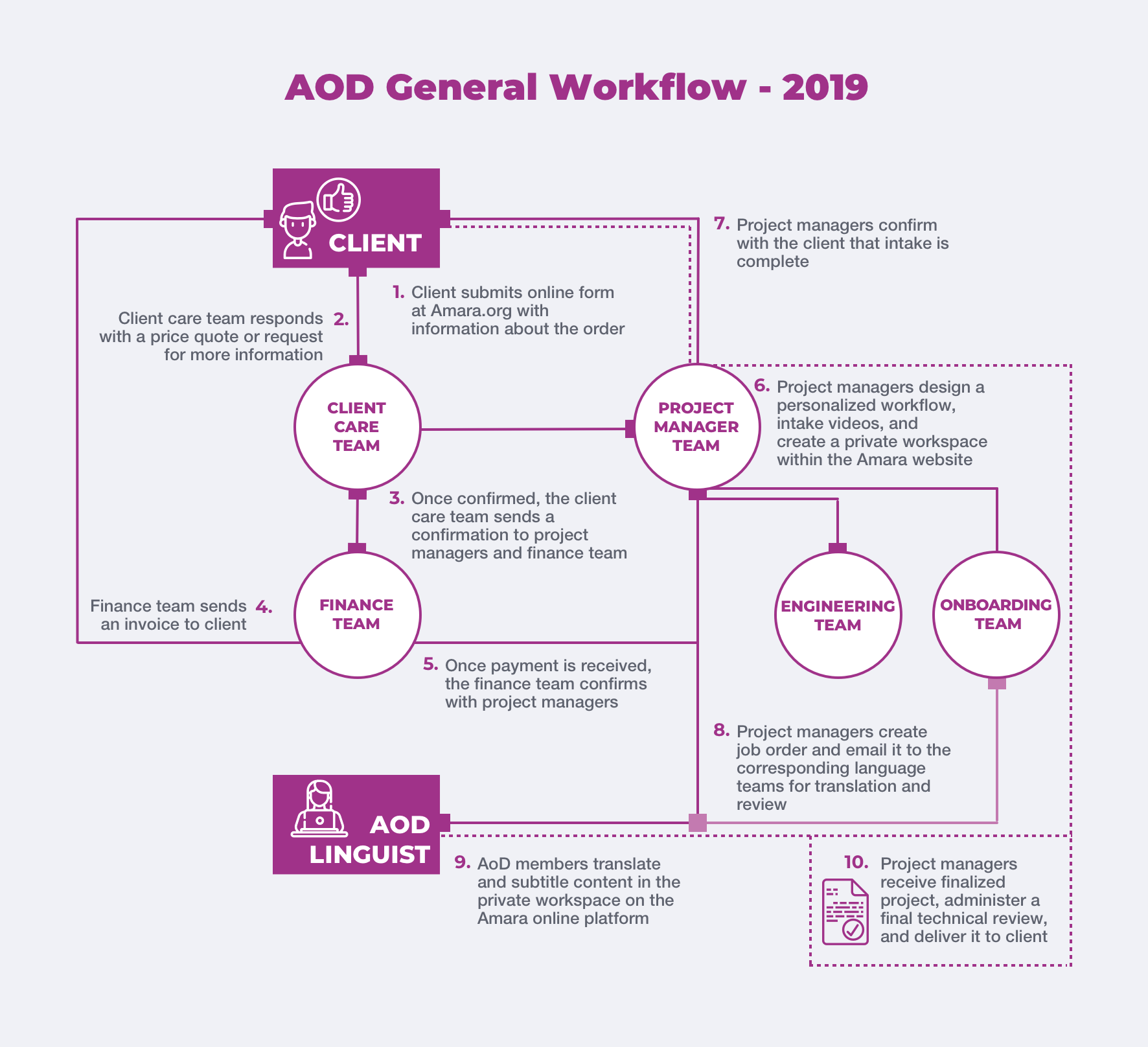
Figure 1 provide an overview of the overall workflow in AOD, as identified and mapped by Zelickson [3] at the time the research was conducted (October 2018 – April 2019).
Evaluating the points of intervention
Drawing on the analysis of this initial set of semi-structured interviews and a literature review on Amara and broader forms of crowdsourcing, we identified several possible “points of intervention” for the development of blockchain-based tools which could potentially help our case study. Among all of them, we finally chose task allocation.
The “first-come, first-served” logic embedded in the platform is the main component of task allocation. This policy creates a competitive dynamic between workers in assigning tasks to themselves. As a result, some linguists find themselves continually checking for work, an issue identified more generally in other crowdsourcing projects [4]. Overall, this logic needs to be understood in an environment with an inconsistent workload.
The results of this analysis led us to define task allocation as our main object of experimentation with blockchain-based tools, with the aim of exploring and implementing new ways to allocate tasks according to communitarian needs. To further our understanding of this point of intervention, we carried out a second stage of data collection and analysis.
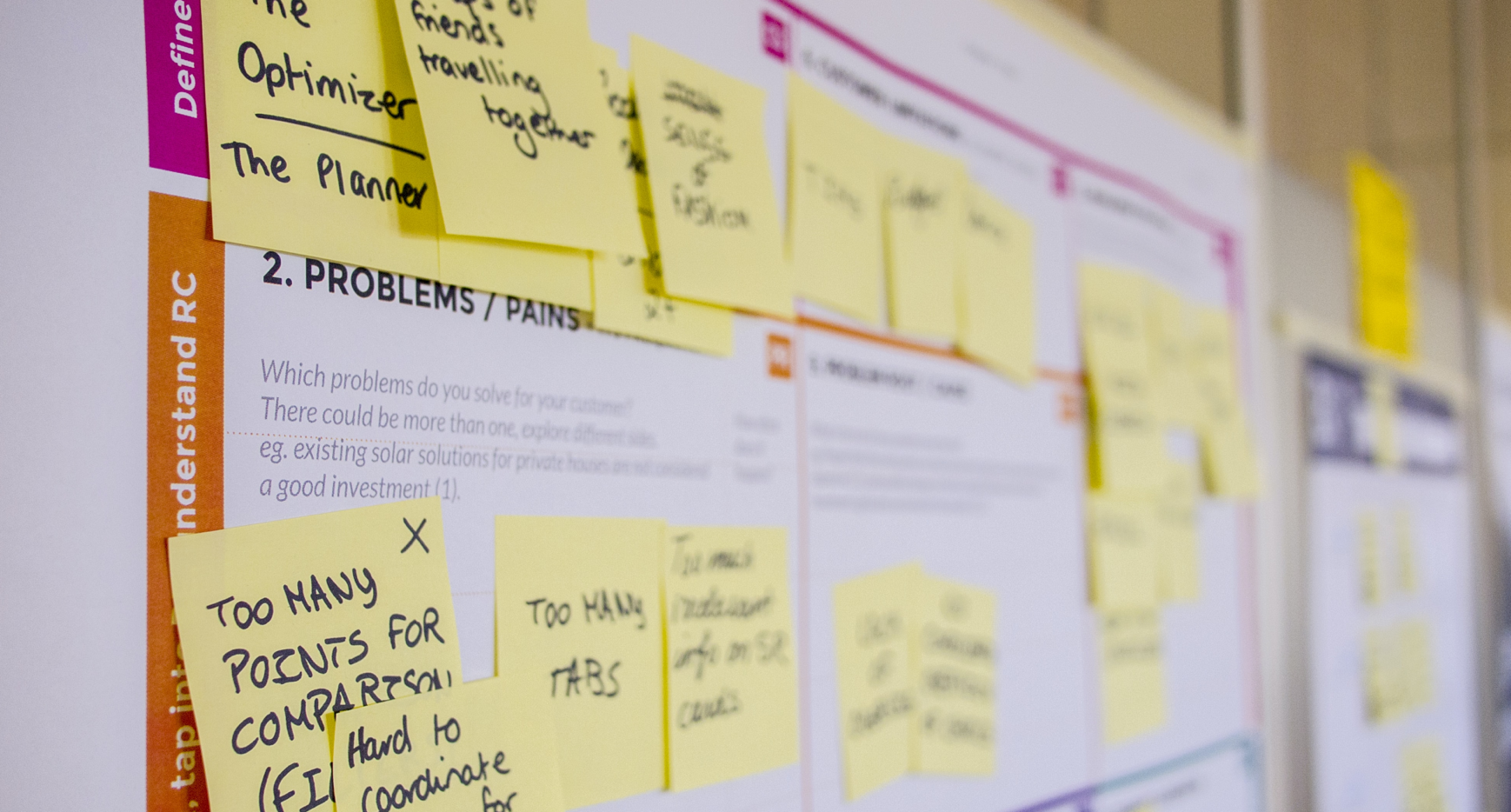
Photo by Daria Nepriakhina on Unsplash
2. As is usual in platform cooperatives and peer production communities, Amara’s workflows and processes are dynamic and constantly evolving.
Stage 2. Prototyping with blockchain
Stage 2 (March 2019 – September 2020) involved a new iteration of data collection and analysis focused on core members of AOD. It involved several months of participant observation, nine semi-structured interviews and documentary analysis of materials generated and posted in the official channels of AOD. This data collection concerned two primary goals. The first was to extend the data concerning organizational processes of the workflow and the changes experienced in it over time. The aim was to include all of the different perspectives of the actors involved in the platform in order to supplement those gathered during stage 1 (focused on linguists). The second goal was to enrich our understanding of the organizational characteristics surrounding the selected point of intervention: task allocation. After analyzing the data, this stage was concluded by organizing a workshop to begin the development of initial prototypes with members of AOD.
Understanding the core of AOD
AOD’s core team facilitates and oversees the whole of the production process, coordinating and sustaining the infrastructure required to achieve the successful creation of subtitles and captioning. The core team operates as a central node in AOD, although their members are globally distributed. During this phase, we carried out participant observation, and conducted semi-structured interviews with key members of AOD’s core team: project managers, developers, members of the finance team, and project leaders, amongst others. We interviewed at least one member of each area, and we decided to explore in even greater depth the vision of project managers. The reason for this was that they are the core team members with the closest contact to our point of intervention: they coordinate each project, prepare and send job orders and monitor overall progress. Having achieved an in-depth picture of the current workflow from the myriad perspectives of AOD members and a richer understanding of the factors surrounding our main point of intervention, stage 2 concluded with the organization of an online focus group to inform, develop and test the initial prototypes.
The first co-designing workshop
The first workshop focused on the identification of alternative task allocation models together with AOD linguists. From all of the groups in AOD (organised by language direction), we chose the Portuguese-Brazilian group as the target one, due to its organizational complexity. The workshop consisted of two parts. The first part involved a two-day online focus group, during which general needs and alternative models for the allocation of tasks were explored. The second part consisted of individual sessions (lasting three days) to carry out user testing with an experimental prototype3. The prototype employed decentralized technologies to embed the same “first-come, first-served” logic for task allocation currently implemented in the official platform. This prototype was aimed at helping to identify the impact and limitations of these decentralized experimental tools before implementing alternative models that emerge from the focus groups. For each part, key insights resulting from the data analysis were as follows:
DISTRIBUTION OF TASKS
We identified three alternative models. These are to be understood as ideal types, whose characteristics can be adapted and combined:

1. ROUND ROBIN
A model characterized by a balanced allocation of work according to the amount of work previously carried out by the linguist. Tasks are offered to linguists during a window of time for acceptance/refusal. If it is rejected, the task is then offered to another linguist.

2. ALLOCATION BY REPUTATION
The tasks are offered to the linguist according to the quality of work done in previous tasks, based on the feedback received by their peers.

3. PRE-SET ASSIGNMENTS ACCORDING TO CONTENT PREFERENCES
Tasks are pre-assigned to the linguist according to their self-reported preferences regarding the content of the videos themselves
The sovereignty for decision-making regarding which models to employ should reside within the language group. Regarding the former, for example, the Brazilian-Portuguese group may decide to work with a Round Robin model. In contrast, the Japanese group may choose to keep on using a “first-come, first-served” model.
Similarly, language groups should be autonomous in deciding the parameters that characterize the selected model. Continuing with the previous example, the Brazilian-Portuguese group should have the autonomy to determine the parameters of their Round Robin model, e.g. how long the window of time is to accept/reject a task, or what the parameters of the workload are. Several models for decision-making (e.g. one person, one vote; plural voting; quadratic voting [15]) could be employed to make collective decisions about these parameters.
Re-allocation of resources between language groups: future blockchain-based interventions could consist of a distribution of resources between different language groups federally. For example, suppose the Brazilian-Portuguese group suffers from a scarcity of work during a certain period. In that case, resources could be allocated from other groups which have had a higher workload during that time.
Specific workshops will be required to refine the parameters for each of the models, as well as to identify more models within different language groups.
User testing of the blockchain prototype
In general, linguists perceive a higher degree of complexity when using the blockchain-based prototype to assign themselves tasks than they do with the current platform. However, they feel a higher degree of ownership, and they were willing to accept this higher degree of complexity to a certain extent.
“Pay for work“: The use of gas (a type of cryptocurrency) to pay for the assignment of the tasks generated uncertainty and was at times rejected. Further research should continue to explore the technical and social aspects surrounding this issue.
The use of a wallet (specifically the Metamask browser plugin) was the key factor in the aforementioned higher degree of perceived complexity. The learning curve for using a wallet is steep at first, although it becomes more comprehensible with time. Precise and clear instructions were critical to being able to carry out testing.
Stage 3. Quantitative analysis and refining the models
Stage 3 (February 2021 – September 2021) involved a new iteration of data collection and analysis. Firstly, this stage focused, drawing on quantitative methods, on furthering our understanding of the overall distribution of work in AOD. Secondly, drawing on qualitative methods, we refined the parameters for the blockchain-based prototypes of the alternative models of task allocation identified during stage 2. Additionally, this stage included a new iteration in the development of blockchain-based prototypes.
Quantitative analysis of AOD’s platform data
Drawing on a collaboration with researchers of Indaga, during this stage, we analysed a dataset comprising variables related to the tasks and the linguists who carried them out. During the period of the study, 336 linguists were active and worked on 29,444 videos, which resulted in 67,415 tasks. The main goal of this analysis was to shed light on the organisational structures and logics of AOD, drawing directly on data automatically collected by the platform. The research involved different types of univariable and multivariable analyses at different levels: tasks, workers and linguistic directions. The results allowed reaching the following four conclusions:
- There are significant differences in the types of tasks and a clear preponderance of transcriptions in contrast to translations.
- There is an uneven distribution in the access of linguists to tasks and the rates applied to them. These distributions typically follow the Pareto Law. Figure 2 below shows, as an example, this distribution considering the total number of tasks.
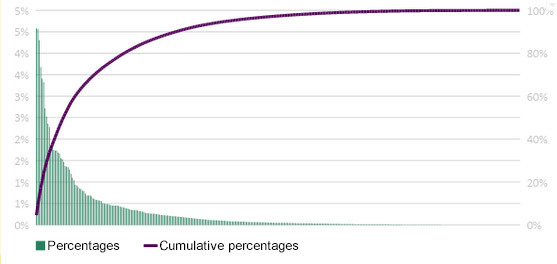
Figure 2. Created by Indaga. Total number of linguists (N= 336)
- The high accumulation degrees identified overall are also significant within the linguistic groups themselves. However, there are notable differences between some of these groups.
- These differences enabled the identification of several language categories from which representative cases for further analysis were selected. Table 1, below, provides an overview of them:
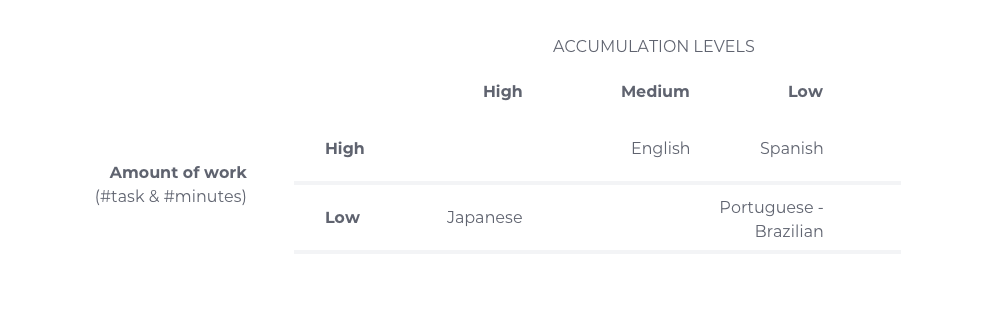
Second workshop with the Portuguese-Brazilian group
This second workshop aimed to identify specific parameters and logics that could be implemented into blockchain-based prototypes. On this occasion, we focused on discussing the three models that emerged from the previous workshop: a circular system for a more balanced distribution of work, a content-based model for distributing tasks, and a reputation model for task distribution.
Session 1: Circular Models
We understand a circular model as one in which work is allocated from a group of eligible resources on a cyclic basis. In terms of Amara, this relates to the capacity of the system to allocate a work item to a selected person chosen from a group of eligible people following a cyclic logic. The circular allocation of tasks allows a more equitable distribution of work. The primary ideas that emerged during this session were:
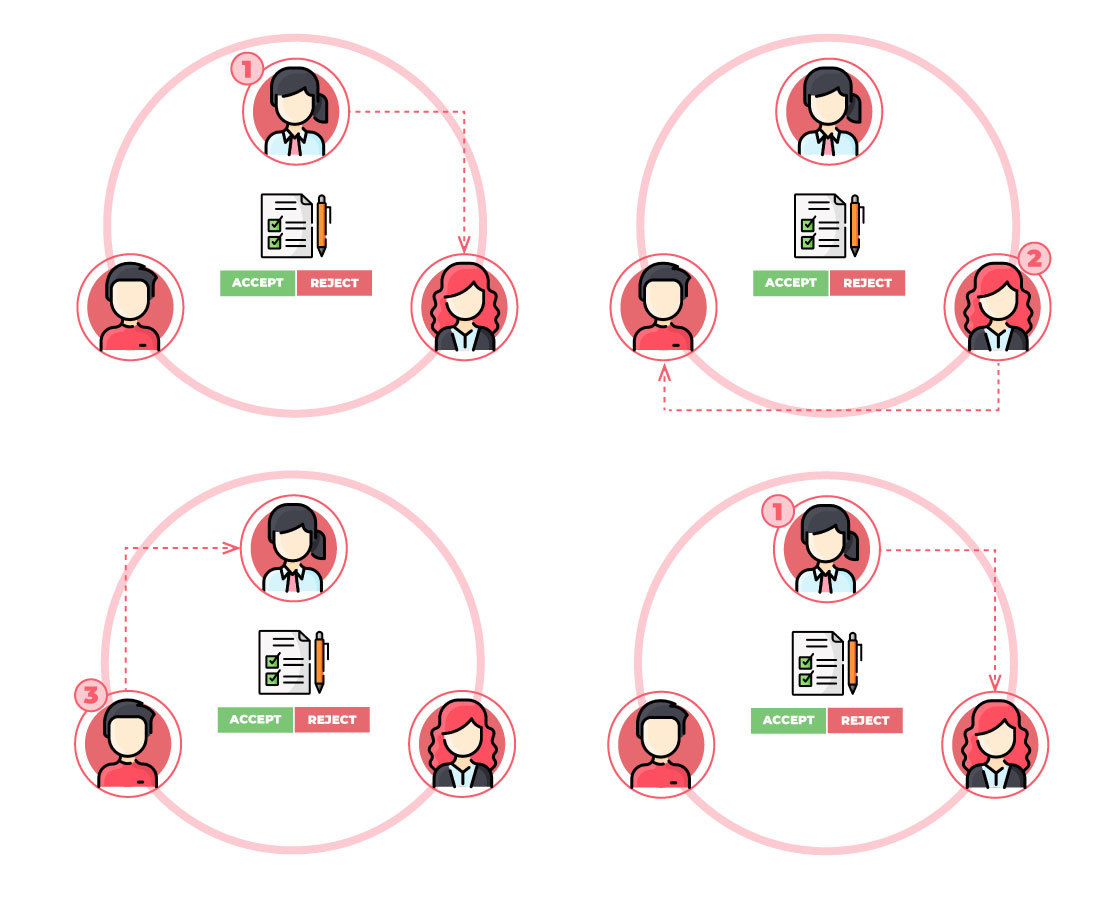
Figure 3. Overview of the circular model
- Linguists should ideally have a way to specify their availability to receive tasks. Two functionalities were discussed here: a calendar or spreadsheet where linguists can indicate their availability and a “switch” to indicate the status (i.e., available, busy, offline, etc.). Both could be combined: the calendar could automatically set the linguist’s status in the platform, while the system could also offer the option to change it on demand.
- The experience of the linguists, understood as the time passed since they joined the platform, should not be taken as the decisive factor to allocate tasks. It can be detrimental to newcomers, and it is also not indicative of the most recent activity. Instead, the number of good translations and subtitles and the time linguists are active on the platform might be more accurate experience indicators.
- The duration of the video should not be the only feature to understand the complexity of the task. In this sense, other characteristics of the videos, such as the type of content (e.g. technical or not) and the amount of text to translate, should be considered to estimate the complexity of tasks.
- As a general rule: complex videos should be allocated to experienced linguists, while more simple videos should be offered to newcomers.
Session 2: Content-based Models
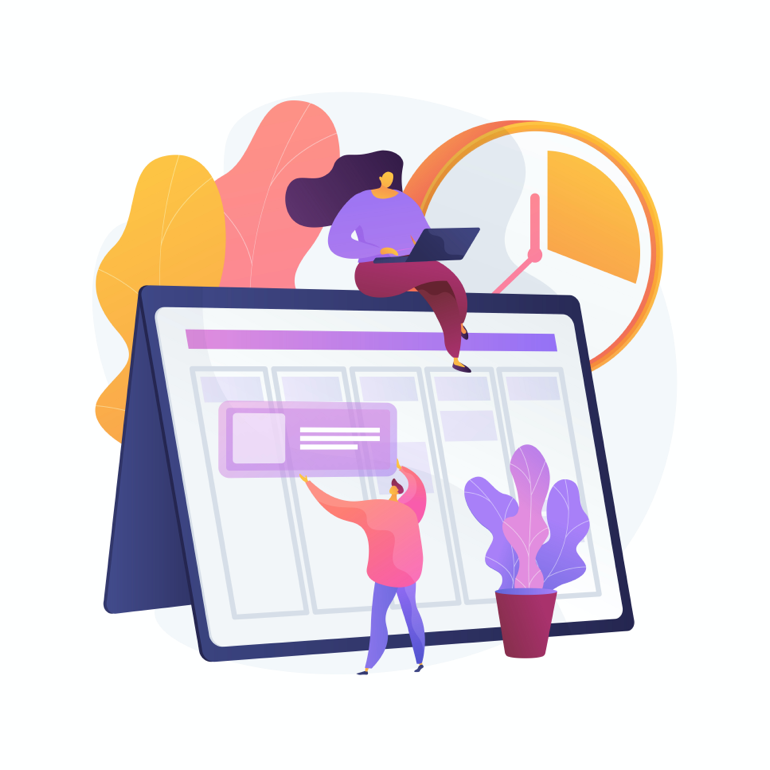
This workshop session focused on discussing how the contents of the videos should be considered in task allocation. In particular, we were interested in understanding if the assignation of tasks should be based on matching the content of the videos with the linguist background and topic preferences (e.g., Maths, Computer Science and Philosophy). Also, we discussed whether it would be helpful to allocate videos whose topics are similar to videos previously translated/subtitled by the linguists. The key ideas that came up during the session were:
- The linguists’ background was not considered particularly useful for the matching. Instead, using linguists’ preferences was indicated as more relevant.
- However, specifying the linguists’ preferences for a successful matching was found to be challenging. Given the broad range of topics, finding a way to precisely indicate these preferences was highlighted as unnecessarily complicated and not relevant enough.
- Due to how Amara operates, by teams rather than by topics, having a model that allocates tasks by topic can be detrimental to the workflow of Amara, so it was also discarded.
- As a result, we concluded content-based specific models should not be explored experimentally. Instead, the content of the videos can be used to parametrise alternative models of task distribution, such as the circular one or the reputation-based.
Session 3: Reputation-based Models
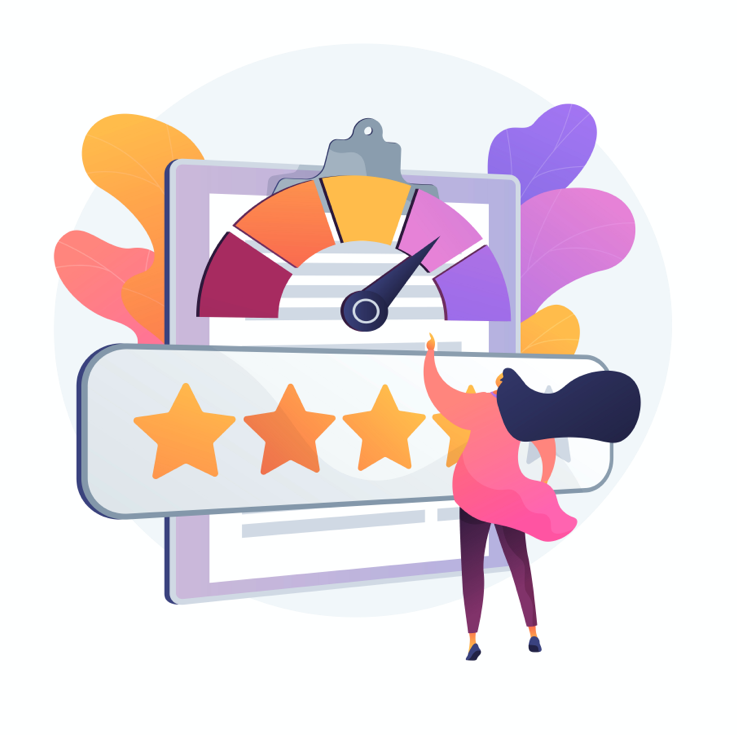
During the session, we discussed building a reputation system based on previous work, attention to deadlines, feedback, past peer reviews, and the type and characteristics of the tasks on which the linguists have worked in the past. The use of levels to classify linguists was also examined in this session. The main ideas that emerged were as follows:
- The linguists’ reputation can be instrumentalised through Badges or points. The assignation of points and badges should consider the characteristics of the tasks carried by the linguists. Some of these characteristics include the duration, whether the video is a technical one or not, and the audio quality. Being attentive to past deadlines was as identified as key for a potential reputation system.
- There could be two types of badges: temporal and absolute. The badges should consider both: the quantity and quality of work.
- Losing points or badges (e.g. after several delays with deadlines) was discussed as a possible feature that considers the needs of the rest of the participants. If framed within a commons-based logic, this could align with Ostrom’s principle of graduated sanctions.
- According to linguists, the main advantage of this model is that it enables one to build a “career” in the platform by progressing through a system with different levels. This reputation-based model should integrate the levels which already operate in the community.
- A system with badges and points could also be employed to incorporate groups’ reputations, not solely individuals.
Development of prototypes
As explained before, in Stage 2 the development team was focused on making two basic prototypes. On the one hand, the “FCFS Model”, which is the one currently being used in Amara, was replicated. On the other hand, a first version of the “Circular Model” was implemented. The stage ended with a workshop in which users of the platform evaluated the usability of the “FCFS Model”. After the recent workshops, the “Circular Model” prototype is being refined and extended based on the suggestions collected during the workshops.
We are using the Ethereum blockchain in the implementation of the prototypes together with Aragon, one of today’s most used frameworks to develop DAOs (Decentralized Autonomous Organizations). Besides, Aragon Connect and The Graph are used to index data stored in the blockchain.
The backend of the prototypes are Smart Contracts written in Solidity. The frontend of the prototypes are built in React with material-ui. We use Aragon Connect to connect the frontend with backend through Aragon. Once the extension of the “Circular Model” is completed, the next step is the development of a reputation-based task distribution model.
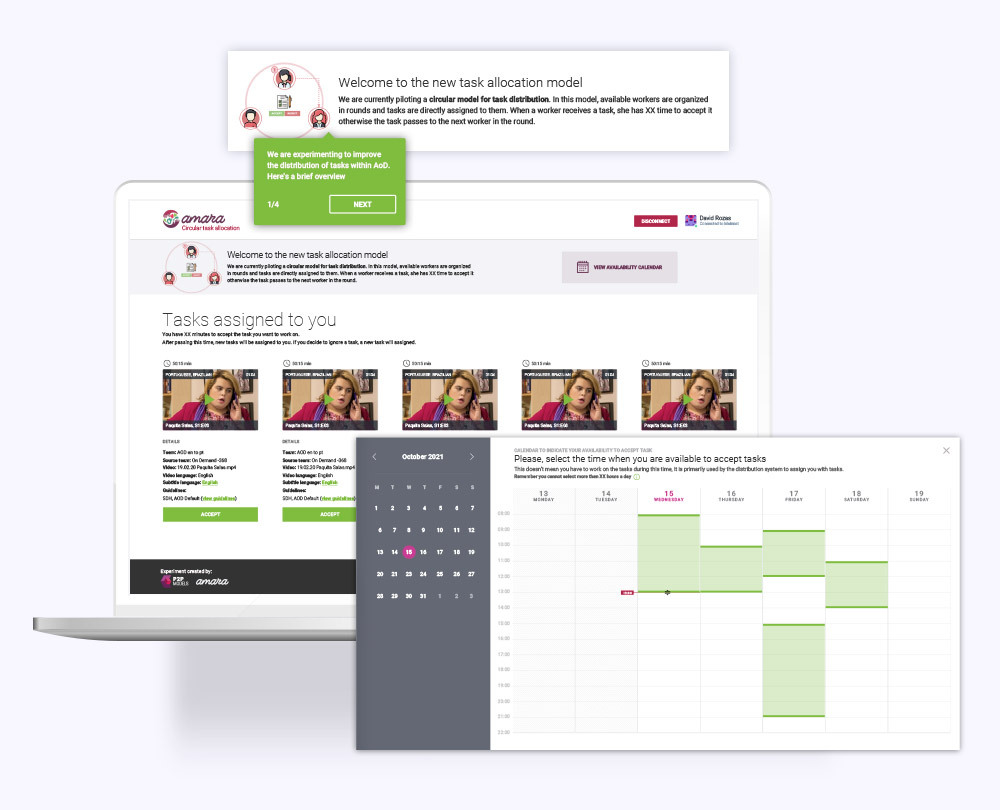
Figure 4. Scheme of the within-group design
Ongoing research and next steps
We will focus our efforts on two lines of research:
- We will continue organising several workshops with different groups selected by language direction and conducting a qualitative analysis of the data generated. The results of our quantitative analysis inform the selection of the specific groups. The next round of workshops will be with the Spanish group.
- We will continue the development of prototypes, considering the parameters identified in the workshops, and develop controlled experiments with them. We aim to compare the performance and the benefits for workers of the models previously identified (see Figure 3).

More information
Related info
References
- Jansen D, Alcala A, Guzman F. Amara: A Sustainable, Global Solution for Accessibility, Powered by Communities of Volunteers. In: Stephanidis C, Antona M, editors. Universal Access in Human-Computer Interaction Design for All and Accessibility Practice. Springer International Publishing; 2014. pp. 401–411.
- Video Translation and Accessibility at Scale – Amara Enterprise. [cited 27 Oct 2020]. Available: https://amara.org/
- Zelickson E. A Balancing Act: Hybrid Organizations in the Collaborative Economy Empirical Evidence from Amara on Demand. University of Brown. 2019.
- Gray M, Suri S. Ghost work: how to stop Silicon Valley from building a new global underclass. Eamon Dolan Books; 2019.
- Faraj S, Jarvenpaa SL, Majchrzak A. Knowledge Collaboration in Online Communities. Organization Science. 2011;22: 1224–1239.
- Harhoff D, Lakhani KR. Revolutionizing Innovation: Users, Communities, and Open Innovation. MIT Press; 2016.
- Amer-Yahia S, Basu-Roy S. Toward Worker-Centric Crowdsourcing. 2016 [cited 31 Jan 2019]. Available: http://sites.computer.org/debull/A16dec/p3.pdf
- Ross J, Irani L, Silberman MS, Zaldivar A, Tomlinson B. Who are the crowdworkers?: shifting demographics in mechanical turk. Proceedings of the 28th of the international conference extended abstracts on Human factors in computing systems – CHI EA ’10. New York, New York, USA: ACM Press; 2010. p. 2863.
- Hansson K, Ludwig T, Aitamurto T. Capitalizing Relationships: Modes of Participation in Crowdsourcing. Comput Support Coop Work. 2018 [cited 31 Jan 2019]. doi:10.1007/s10606-018-9341-1
- Hansson K, Aitamurto T, Ludwig T. From alienation to relation: Examining the modes of production in crowdsourcing. 2017 [cited 31 Jan 2019]. doi:10.18420/ecscw2017-13
- López LE. Plan de Ayala: Un siglo después. Instituto Nacional de Antropología e Historia; 2018.
- Mcneely JH. Origins of the Zapata Revolt in Morelos. Hisp Am Hist Rev. 1966;46: 153–169.
- Scholz T. Platform Cooperativism. Challenging the Corporate Sharing Economy. 2016.
- Rozas D, Tenorio-Fornés A, Díaz-Molina S, Hassan S. When Ostrom Meets Blockchain: Exploring the Potentials of Blockchain for Commons Governance. Sage Open. 2021;(in production). doi:10.1177/21582440211002526
- Cossar S, Berman P. Democracy Earth Foundation Digital Decision-Making Pilots Report. Democracy Earth; 2020 Oct. Available: https://www.dropbox.com/s/udcg0og64wlcqft/Democracy%20Earth%20Pilots%20Report.pdf?dl=0
You must be logged in to post a comment.