Today, digital platforms are increasingly mediating our day-to-day work and crowdsourced forms of labor are gaining importance. Platforms like Amazon Mechanical Turk and Universal Human Relevance System taskify work and draw on a volatile and distributed crowd of workers that compete to find their next paid task. We are exploring alternative models to task allocation logics defined and agreed upon by the workers of the platforms, so the platforms belong to those who work on it.
These platforms operate as labor marketplaces for businesses to outsource work to globally distributed and diverse workers. Tasks are carried out by an invisible workforce of “humans in the loop”. The logic behind the allocation of these tasks typically operates on a “First-Come, First-Served” (FCFS) basis. This generates a competitive dynamic in which workers are constantly forced to check for new tasks, producing a sense of anxiety and frustration if they do not obtain work. The FCFS logic also tends to create inequitable work distribution, by relying on circumstances that are often beyond workers’ control.
“
Alternative allocation tasks models involve the producers progressively becoming owners of the means of production and the fruits of their labor expressions of self-realization and, as a result, platforms might belong to those who work on them.
An alternative to competitive dynamics
There is a lack of alternative models which aim to improve the working conditions and wellbeing of workers. This is particularly true when it comes to approaches involving co-designing with the workers themselves. Here we present the preliminary results of an iterative design approach where workers have been involved throughout a research process that included a variety of methods. Our aim is to identify and validate alternative task allocation logics defined and agreed upon by the workers of the platforms.
Our vision towards more worker-centric crowdsourcing platforms is summarized by the motto: “the platform belongs to those who work on it”. The aim is to increase the degree of workers’ power in crowdsourcing platforms: the means of production. To this end, we have identified, with crowdsourcing workers, three alternative models of task allocation beyond “First-Come, First-Served”, namely (1) round-robin, (2) reputation-based, and (3) content-based. In our ongoing research, we will experiment to see whether these models could create fairer and more collaborative forms of crowd labor.
Karl Marx has something to say here
Workers’ relationships with the platform can be described as alienating. Hansson et al. in their work Capitalizing Relationships: Modes of Participation in Crowdsourcing drew on Marx’s theory of alienation to understand the relationships between participants in crowdsourcing and the role of the platforms employed to mediate activities. They developed a typology of alienation that reveals significant differences between the cases studied. The graphic below compares Amazon Mechanical Turk and our main case study in this research, Amara. The farther from the center, the higher the degree of alienation.
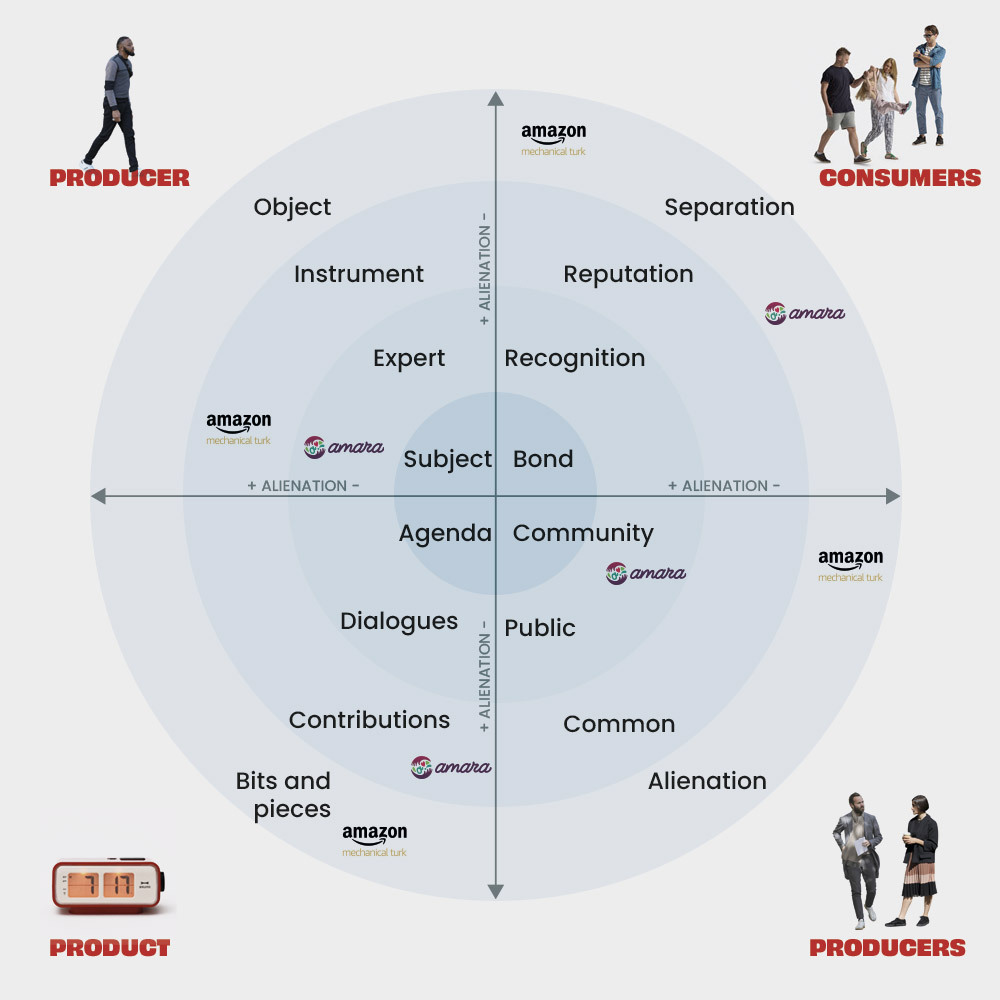
Following Hansson et al.’s work, and to further our understanding of how crowdsourcing platforms might support social relationships in these contexts, instead of merely capitalizing them, we established a collaboration with Amara on Demand (AOD). AOD is a collaborative platform for the creation of subtitles as an on-demand paid service which is inspired by cooperative and commoning practices. It shows a remarkable contrast to the market-based logic of other crowdsourcing platforms, such as Amazon’s. Over the years, AOD moved from a few linguists to more than nine hundred at the time of writing. The work of linguists in AOD is remunerated and they are organized on a per-language direction basis. AOD employs a FCFS model for the distribution of tasks, but they are open to explore more cooperative ways of distributing work. We decided to explore the following research question with them: Can we identify alternative models for the distribution of tasks in crowdsourcing that consider the needs of the workers?
Methodology of the study
Principal characteristics of the study
If you want to know the methods used to arrive at our conclusions, please unfold the table below
Research question
Can we identify alternative models for the distribution of tasks in crowdsourcing that consider the needs of workers?
Approach
Multi-modal qualitative approach that combines different methods
Methods
25 online and face-to-face (F2F) semi-structured interviews: 17 to linguists and 8 to core members of AOD
Focus groups (June 2020)
Documentary analysis of 55 documents, mainly internal AOD documents provided to linguists and official blog posts
Online participant observation carried out over six months (October 2018 – March 2019)
Measured variables
Gender, main role in AOD, number of years in AOD. Also, for participants in F2F interviews and focus groups: location and language groups they belong to
Point of
intervention
The analysis of these data led us to work on alternative models for task allocation as they represent a suitable starting point for envisioning more cooperative labor processes
Current model of task distribution in AOD: FCFS
This logic creates a competitive dynamic between linguists to assign tasks to themselves. The competitiveness embedded in this logic becomes even more problematic in a global environment, as in the case of AOD. For example, in theory all linguists belonging to the same language group should have the same opportunity to assign themselves a specific task. However, the reality is that some of them might be in time zones that are less convenient concerning the times at which tasks are usually posted. Overall, FCFS encompasses a need for workers to check continuously to find more work, an issue also identified in other crowdsourcing projects. Some linguists also suggested that the competitiveness embedded in the platform’s task allocation method undermines the sense of community.
Alternatives to the FCFS model
Round-Robin (RR)
This model refers, in computer science, to an algorithm proposed in the context of operating systems to decide how to schedule multiple processes competing simultaneously for CPU time: the amount of time employed to process computer instructions. It is one of the simplest and most straightforward solutions to avoid starvation in process execution and it is known as one of the fairest scheduling algorithms. The model can be thought of as circular, in which tasks are distributed in a balanced way.
The main advantage discussed by the linguists regarding this model is that it tackles the competitive character.
The challenge of this model lies in identifying the specific parameters to encode in these forms of RR allocation and in providing the linguists with mechanisms that enable them to reach a consensus among themselves.
Reputation based model
Reputation systems have been proposed to build trust among Internet users. They are based on collected and aggregated feedback about users’ past work and help to foster trustworthy behaviors, assess credibility, and discourage dishonest participation. In the context of AOD, this category emerged as a model in which tasks are offered to linguists according to the quality and quantity of previous work, based on feedback received by their peers, and depending on the characteristics of the tasks themselves.
A problem tackled by this model, according to the linguists, is that it increases transparency concerning the processes by which they are promoted on the platform. It could also help to tackle the need to constantly check for work.
The challenge of a reputation-based model, as that proposed by the linguists, is that of arriving at agreements on what to consider within the system. However, within the focus group, linguists reached a preliminary consensus regarding its application in the context of the current AOD levels system. The system proposed was based on the explicitation of the parameters which already operate for promotion between the different tiers on the platform.
Content-based model
Recommendation systems are the cornerstones of modern online services. In social media, they are used to suggest publications, in e-commerce sites to offer products, in video-streaming applications to recommend multimedia materials, and in crowdsourcing platforms to suggest tasks to workers. They focus on matching the characteristics of the artefact to recommend (e.g. topic of publication, movie genre or task description) with users’ attributes based on their profiles and historical data.
For our case study, this model emerged as one in which tasks could be pre-assigned according to two different types of possible matching logics: (1) either the linguist’s skills and/or personal preferences regarding specific areas of knowledge, and (2) the linguist’s previous experience on the platform concerning the complexity and/or size of the task.
A task allocation model that includes features of the tasks (e.g. complexity, size, topic, length) and workers’ skills, background, and preferences was also seen by the participants as a suitable complement to reputation systems. Furthermore, this method has been found to work well to match workers with tasks relevant to their expertise and skills, resulting in high quality work.
Conclusion
Despite the inherent limitations due to the ongoing nature of this research, the models identified throughout the collaboration with AOD show us that it is possible to envision more cooperative models to distribute work. These models involve the producers progressively becoming owners of the means of production and the fruits of their labor expressions of self-realization and, as a result, platforms might belong to those who work on them.
Furthermore, we want to explore how to develop tools which allow crowdsourcing workers to decide on the models to use in different contexts. Similarly, these tools to support decision making could be employed to collectively determine how a model could be parameterized. In doing so, we plan to develop collaborative decision making tools that leverage the affordances of distributed ledger technologies, such as blockchains, to allow crowdsourcing workers to prioritize parameters within the models which better suit their needs, as well as to decide collectively between the models themselves.
You can find further details of this ongoing research in the following paper.
SHARE
AUTHOR
Authorship is by Genoveva López, but this content has been made by the whole P2PModels team
Images are by Elena Martínez
Review by David Rozas
Copy editing is by Tabitha Whittall
Rosa Chamorro and Samer Hassan make everything possible